Doctors Go to Jail. Engineers Don’t.
Welcome to the dystopia of AI in healthcare. Where the real innovation happens in billing software while life-saving tech remains a pipe dream. Let’s talk about why.
Welcome to AI Health Uncut, a brutally honest newsletter on AI, innovation, and the state of the healthcare market. If you’d like to sign up to receive issues over email, you can do so here.
Note: This article was also published in The American Journal of Healthcare Strategy, where I was invited to contribute.
Let me ask you this: When you think of AI advancements in healthcare, what’s making the most noise?
From where I sit, it’s the “low-hanging fruit” of AI—the no-risk, zero-liability zones like AI scribing and the so-called “Rev Cycle” management. These non-clinical areas are getting all the hype, as I detail in my digital health industry review of 2024.
But when it comes to clinical progress—where doctors could actually use AI as a “second opinion” tool—we’re seeing a whole lot of nothing.
Why is that?
This is the number one problem and the tragedy of AI adoption in medicine:
Until the liabilities and responsibilities of AI models for medicine are clearly spelled out via regulation or a ruling, the default assumption of any doctor is that if AI makes an error, the doctor is liable for that error, not the AI.
It’s important to point out that if AI makes an error, but the recommendation aligns with the “standard of care,” the doctor may not be held liable for that mistake. So, the real question is:
Until AI itself becomes the standard of care, why would doctors and hospitals pour money and resources into AI systems, when the minimal liability risk is to stick with the existing standard of care?
A growing number of academic studies are addressing the critical issue of physician liability when AI makes an error:
1️⃣ Koppel R, Kreda D. Health Care Information Technology Vendors’ “Hold Harmless” Clause: Implications for Patients and Clinicians. JAMA. 2009;301(12):1276–1278. doi:10.1001/jama.2009.398. IT vendors routinely use “hold-harmless” clauses in contracts with physicians to shift the burden of liability onto healthcare providers, even when the error stems from flaws in the IT system or software.
2️⃣ Froomkin AM, Kerr I, Pineau J. When AIs outperform doctors: confronting the challenges of a tort-induced over-reliance on machine learning. Ariz Law Rev 2019;61:34 99. When AI-based technology becomes the standard of care, the liability of medical providers for failing to adopt it, especially when it could have prevented harm to a patient, can be significant under malpractice law. Both hospitals and treating physicians could face negligence claims if they rely solely on human judgment rather than utilizing superior AI models, particularly in situations where the AI could have led to better patient outcomes. I discuss this scenario in detail in my recent article, “What if a Physician Doesn’t Use AI and Something Bad Happens?” following the November 29, 2023, congressional hearing, “Understanding How AI is Changing Health Care.”
3️⃣ Gerke S, Minssen T, Cohen G. Ethical and legal challenges of artificial intelligence-driven healthcare. Artificial Intelligence in Healthcare. 2020:295–336. doi: 10.1016/B978-0-12-818438-7.00012-5. Epub 2020 Jun 26. PMCID: PMC7332220. AI-driven clinical decision support (CDS) tools are currently considered as aids under the control of healthcare professionals. Therefore, even if an AI system provides an incorrect recommendation, the clinician could be held responsible under medical malpractice law because they are expected to use their expertise to make the final decision. AI tools are generally regarded as supplementary, and clinicians are seen as the “captain of the ship,” retaining ultimate responsibility for decisions. The article also raises the possibility of product liability claims against AI developers but notes that such cases have been difficult to win because courts often view the AI as a tool for clinical use rather than an independent decision-maker.
4️⃣ Griffin, Frank, Artificial Intelligence and Liability in Health Care (May 21, 2021). 31 Health Matrix: Journal of Law-Medicine 65-106. Doctors are responsible for ensuring that AI outputs are interpreted correctly and integrated properly into patient care. If a physician relies solely on AI without using clinical judgment, they might be liable for malpractice. The physician’s duty does not change simply because AI is involved. Rather, their role as the intermediary between the AI and the patient becomes more critical.
5️⃣ Maliha G, Gerke S, Cohen IG, Parikh RB. Artificial Intelligence and Liability in Medicine: Balancing Safety and Innovation. Milbank Q. 2021 Sep;99(3):629-647. doi: 10.1111/1468-0009.12504. Epub 2021 Apr 6. PMID: 33822422; PMCID: PMC8452365. Medical professionals may be held responsible for errors made by AI systems because they have a duty to independently evaluate AI recommendations and ensure they meet the standard of care. Courts have historically held physicians liable when relying on faulty medical literature or devices, suggesting that doctors could face liability if they blindly follow AI outputs without applying their own clinical judgment. The article emphasizes that policy should focus on balancing liability across the ecosystem, including developers, health systems, and clinicians.
6️⃣ Price, W. Nicholson; Gerke, Sara; and Cohen, I. Glenn, Liability for Use of Artificial Intelligence in Medicine (2022). Law & Economics Working Papers. 241. Medical professionals using AI could bear responsibility for errors made by AI systems, particularly if the AI’s recommendations deviate from the standard of care and cause harm. However, this area of law is still developing, and future cases and regulations will likely clarify these responsibilities further.
7️⃣ Mello MM, Guha N. Understanding Liability Risk from Using Health Care Artificial Intelligence Tools. N Engl J Med. 2024 Jan 18;390(3):271-278. doi: 10.1056/NEJMhle2308901. PMID: 38231630. When physicians rely on AI outputs for patient care, they can still be held liable for malpractice if an error leads to patient harm. Courts evaluate whether a physician’s decision to follow or reject an AI model’s recommendation aligns with what a reasonable specialist would have done in similar circumstances. For example, physicians must critically assess the reliability of AI outputs, particularly if there are known issues such as ‘distribution shift’ (when the AI’s training data do not match the patient population). (My loyal readers already know that I flagged ‘distribution shift’ as obstacle #2 in my recent analysis of the painfully slow AI adoption in medicine. It’s reassuring to see that Stanford researchers and The New England Journal of Medicine are on the same page. 😉) The article also notes that tort doctrine is still evolving in this area. There is no clear precedent on how to allocate liability between AI developers and medical professionals. Courts may treat AI similarly to older decision-support tools, focusing on whether the physician should have known not to follow the AI’s recommendation based on available evidence.
8️⃣ Walsh, Dylan, Who’s at Fault When AI Fails in Health Care? Stanford HAI, 24 Mar. 2024, https://hai.stanford.edu/news/whos-fault-when-ai-fails-health-care. Accessed 8 Sept. 2024. Professor Michelle Mello of Stanford offers four key recommendations on mitigating AI liability risks in medicine. First, hospitals should thoroughly assess and vet AI tools before using them. Second, they must document how AI is used in patient care. Third, it’s critical to negotiate liability terms with AI vendors, being cautious of “hold-harmless” clauses that shift responsibility to medical providers. Finally, hospitals should consider disclosing AI use to patients to foster transparency and trust.
This is the most outrageous double standard. If someone dies, it’s the medical provider who is liable for that human life, not a machine learning engineer who made a modeling error that contributed to a misdiagnosis. AI ethics and AI responsibility standards must change!
Until then, the default rule and default fear of the medical community will be: Doctors go to jail. Engineers don’t.
Why would anyone go to jail for following a well-validated and widely industry-tested AI model? Because if, God forbid, your patient dies and the AI’s recommendation falls outside the norms of the “standard of care,” you’d be in violation of malpractice laws. Doctors have faced criminal charges for malpractice for decades, with some sentenced to as many as 20 years in prison. (For example, see the case of Dr. Robert Levy.) On the flip side, doctors can also go to jail for saving a life if their actions fall outside the “standard of care.” I know one doctor from Upstate New York who gave a patient an experimental drug that wasn’t FDA-approved at the time. He believed, based on his professional judgment and the Hippocratic Oath, that it was the only way to save the patient’s life. Nonetheless, he was sentenced to 34 months in federal prison. Here is a wild twist. While he was in prison, the drug was approved by the FDA. But it didn’t matter—he still served the full sentence.
I’ve scrutinized guidelines from five leading organizations on using AI in healthcare: OECD, WHO, FDA, AMA, and AAFP. (Source: Polevikov S. Advancing AI in healthcare: A comprehensive review of best practices. Clin Chim Acta. 2023 Aug 1;548:117519. doi: 10.1016/j.cca.2023.117519. Epub 2023 Aug 16. PMID: 37595864.) Not one of them addresses the critical issue of liability when AI makes an error.
Health tech companies, hospitals, and even the American Medical Association (AMA), whose primary mission is to advocate for physicians and patients, have shifted much of the burden onto physicians. They argue that “doctors making the final call in care are ultimately responsible for their decisions”. (Source: Politico.) The OECD, WHO, FDA, and AAFP are silently nodding in agreement. (Source: Polevikov S. Advancing AI in healthcare: A comprehensive review of best practices. Clin Chim Acta. 2023 Aug 1;548:117519. doi: 10.1016/j.cca.2023.117519. Epub 2023 Aug 16. PMID: 37595864.) To add insult to injury, the AMA has been playing both sides, flip-flopping on AI liability. They argue that doctors should have limited liability, yet still insist that physicians are the ones holding the bag as the final decision-makers. (Sources: AMA AI Principles, Politico.)
“Physicians are the ones that kind of get left holding the bag.” —Michelle Mello, a Stanford health law scholar.
If we want physicians to accept AI, we must also clarify the physician’s role as an AI prompt engineer. Legal issues need to be addressed. (Source: Anjali Bajaj Dooley Esq., MBA on LinkedIn.)
Another big issue is that
Tech companies selling AI models for medicine couldn’t care less about medicine.
Here is another issue:
Administrators, not medical professionals, are the ones evaluating AI algorithms.
(Sources: “A Clinician’s Wishlist for Healthcare AI Model Evaluations by Sarah Gebauer, MD, “Organizational Governance of Emerging Technologies: AI Adoption in Healthcare”)
Never buy an AI that doesn’t have documentation supporting the seller’s claims about its capabilities!
The problem is that AI models for medicine are often developed by people who know nothing about medicine. They just grab a dataset, train their models on it, publish their paper, and move on with their lives. (For example, see “Med-Gemini by Google: A Boon for Researchers, A Bane for Doctors.”)
As Mayo Clinic and HCA quickly found out, off-the-shelf models built by those who know nothing about healthcare create a dangerous ethical precedent.
(Source: “Painful AI Adoption in Medicine: 10 Obstacles to Overcome.”)
Instead, we must establish a culture of responsible machine learning in medicine.
In particular, IT organizations must adopt the principles of AI ethics and responsibility:
✅ OECD’s Principles on Artificial Intelligence, the first international standards agreed upon by governments for the responsible stewardship of trustworthy AI (2019).
✅ WHO’s 6 Core Principles of Ethics & Governance of Artificial Intelligence for Health (2021).
✅ FDA’s 10 guiding principles that inform the development of Good Machine Learning Practice (GMLP) (2021).
✅ AMA’s principles and recommendations concerning the benefits and potential unforeseen consequences of relying on AI-generated medical advice and content that may not be validated, accurate, or appropriate (2023).
✅ AAFP’s Ethical Application of Artificial Intelligence in Family Medicine (2023).
✅ My recent paper boils it down to the fact that those who build models should ensure AI models are ethical and responsible (2023).
If another IT vendor reaches out to you for a demo, ask them how they are compliant with these principles of AI ethics, AI safety, and AI responsibility. For the sake of your patients, don’t take any chances.
In the U.S., California’s new AI bill, SB 1047, not only failed to address the issue of AI liability but actually extended more protections to AI developers for making errors. The bill softens legal consequences for developers when their AI models cause harm or fail to perform as expected. Essentially, it shields developers by reducing their responsibility in cases where their AI systems malfunction or lead to adverse outcomes, making it more difficult to hold them accountable or pursue legal action. This relaxation might include creating exemptions, lowering penalties, or adding conditions that allow developers to avoid liability. Developers are legally liable for damages caused by their models only if they neglect to implement precautionary measures or commit perjury in reporting the model’s capabilities or incidents. So, if a physician uses an AI model and it harms a patient, liability would likely fall on the physician rather than the AI developer. (Source: Bulletin of the Atomic Scientists.)
What about Europe? The land of GDPR and regulators with a pulse—are they any better at writing laws that actually hold AI developers accountable when their algorithms screw up in medicine?
What Lessons Can the U.S. Learn from the EU’s AI Regulations?
While many believe the EU is more “advanced” in regulating AI than the U.S., I’d be cautious about using the word “advanced.” Yes, the EU was more proactive by approving the first draft of the AI Act last year, but the final draft has yet to be adopted. Even when it is, it may not be the model everyone hopes for. There is widespread consensus among data scientists, startup founders, investors, and policymakers in the U.S. that the country’s lead in AI advancement might be due to less regulation, not more.
We must weight weigh our options carefully. In recent years, a sense of distrust and apprehension has grown within the medical community toward new technologies, partly due to frauds and get-rich-quick schemes like Theranos, Babylon Health, Outcome Health, Bright Health, CareRev, Health IQ, and others. (Source: 10 Lessons from 30 Recent Digital Health Failures. Some May Surprise You.) There’s concern that excessive governance and regulation could prevent pivotal AI advancements from ever reaching clinicians and patients. Striking the right balance, while ensuring protection for both clinicians and patients, is crucial.
Regardless of differing opinions, it’s worth examining the issues the EU has addressed regarding AI liability in medicine.
Specifically, the EU has been proactive in addressing AI liability through regulations like the AI Liability Directive and the EU AI Act. Here are some lessons the U.S. could take from the EU’s approach:
1️⃣ Clear Liability Framework: The EU’s AI Liability Directive aims to modernize liability rules to include AI systems, ensuring that victims of AI-related harm have the same level of protection as those harmed by other technologies. This directive introduces a rebuttable presumption of causality, easing the burden of proof for victims. (Source: The European Commission’s AI Liability Directive.)
2️⃣ Human Oversight: The EU AI Act emphasizes the importance of human oversight in high-risk AI systems, particularly in healthcare. This ensures that AI tools are used responsibly and that there is a clear chain of accountability. (Source: Harvard University’s blog “From Regulation to Innovation: The Impact of the EU AI Act on XR and AI in Healthcare.”)
3️⃣ Transparency and Accountability: The EU’s regulations require detailed record-keeping, robust data quality, and clear user information to ensure transparency and accountability. This helps in identifying the source of errors and determining liability. (Source: Harvard University’s blog “From Regulation to Innovation: The Impact of the EU AI Act on XR and AI in Healthcare.”)
4️⃣ Risk-Based Classification: The EU AI Act classifies AI systems based on their risk levels, with stringent safety measures for high-risk systems. This approach ensures that the most critical applications, like those in healthcare, are subject to rigorous standards. (Source: Harvard University’s blog “From Regulation to Innovation: The Impact of the EU AI Act on XR and AI in Healthcare.”)
5️⃣ Disclosure of Evidence: The AI Liability Directive gives national courts the power to order the disclosure of evidence about high-risk AI systems suspected of causing damage. This can help in establishing liability and ensuring that victims receive compensation.(Source: The European Commission’s AI Liability Directive.)
By learning from these European lessons, the U.S. could develop a more robust framework for determining liability in cases where AI errors negatively impact patients. This would establish clearer guidelines for physicians, hospitals, and AI developers, ensuring accountability for all parties and making sure that AI is truly helping patients, not harming them.
For the AI to start helping doctors, we first must understand
Why Doctors Don’t Trust AI:
1️⃣ Nature of Work and Decision Making: Medical decision-making is complex and individualized. Each patient’s case is unique, and AI might not always capture the nuances of human biology and disease. Doctors might be skeptical about relying on AI for diagnoses and treatment recommendations. (Source: “Why Do Cops Trust AI, But Doctors Don’t?”)
2️⃣ Consequences of Errors: In medicine, the stakes are incredibly high. A misdiagnosis or incorrect treatment can directly lead to severe health consequences or death. Doctors may be more cautious about trusting AI due to the potential for harm. They take their Hippocratic Oath very seriously. Here is a problem though, as I mentioned earlier, and one that’s also a concern among both members of Congress and academics: “What if a Physician Doesn’t Use AI and Something Bad Happens?” Where is the Hippocratic Oath now? It’s a hard conversation, but we’re at a point where physicians cannot afford not to use AI. (Source: “Why Do Cops Trust AI, But Doctors Don’t?”)
3️⃣ Data Quality and Availability: While medical data is abundant, it’s often fragmented, inconsistent, and subject to strict privacy regulations. The quality and comprehensiveness of medical data can limit the effectiveness of AI in healthcare. (Source: “Why Do Cops Trust AI, But Doctors Don’t?”)
4️⃣ Regulatory and Ethical Considerations: The healthcare industry is heavily regulated, with significant ethical considerations around using AI. There’s a higher burden of proof for safety and efficacy before AI tools can be adopted in clinical settings. No matter how good the AI is, human doctors remain the final decision-makers on patient health, which is an enormous responsibility. (Source: “Why Do Cops Trust AI, But Doctors Don’t?”)
5️⃣ Make an AI model intuitive enough that I don’t need to do another online learning session: While many doctors are tech-savvy, the medical curriculum traditionally emphasizes human judgment and experience over technological solutions, contributing to slower adoption and trust in AI. Creating AI tools for doctors that can be used quickly, without a high ‘start-up cost’ every time they use it, will improve care all around. (Sources: “A Clinician's Wishlist for Healthcare AI Model Evaluations by Sarah Gebauer, MD, “Organizational Governance of Emerging Technologies: AI Adoption in Healthcare”.)
6️⃣ Transparency and Explainability of AI: Medical professionals often require high level of transparency and need to understand how AI reaches its conclusions to trust its recommendations, especially given the complexity of human health. As I allude to in one of my forthcoming articles, the explainability of AI is a myth. Currently, doctors must choose between AI complexity and performance, or lack of accuracy but more “explainability.” Unfortunately, you can’t have both. (Source: “Why Do Cops Trust AI, But Doctors Don’t?”)
7️⃣ Provide Metrics That Physicians Understand: Physicians are cautious about using tools that may be biased or provide inaccurate information in certain groups. Present this information in straightforward language, avoiding technical jargon like ‘AUC’ (Area Under the Curve). The proposed model cards are a great starting point, but they should be made even more user-friendly and clear. Prioritizing this will ensure that healthcare AI tools are used correctly in the right patients and settings. This approach benefits patients and ultimately rewards AI developers by ensuring the product performs as intended—because a reliable product is simply good business. (Sources: “A Clinician’s Wishlist for Healthcare AI Model Evaluations by Sarah Gebauer, MD, “Organizational Governance of Emerging Technologies: AI Adoption in Healthcare”.)
8️⃣ Tell Physicians Clearly How the AI Model Compares to Baseline: When evaluating AI models, benchmarks are a key tool for comparing performance. The baseline might be the success rate of current practices or established guidelines. Many clinical AI tools use these guidelines as their baseline. If that’s the case, make it clear, as this will reassure physicians who are more comfortable relying on guidelines rather than the opaque nature of AI. If the AI model doesn’t follow a guideline, explicitly inform physicians where and how it deviates from accepted specialty guidelines. Physicians are legally responsible for the actions they take based on AI recommendations, so don’t force them to sift through papers to justify decisions that diverge from standard clinical practice. (Sources: “A Clinician’s Wishlist for Healthcare AI Model Evaluations by Sarah Gebauer, MD, “Organizational Governance of Emerging Technologies: AI Adoption in Healthcare”.)
9️⃣ Explain to Physicians the Difference Between AI models in Theory vs Real-World Application: Many tools that seem promising in theory often underperform in practice. Early studies on AI’s impact on radiology diagnostic accuracy highlight that the way AI is integrated into workflows is as crucial—if not more so—than its capacity to deliver correct answers. As the field of human-AI interaction evolves, we can expect better data to guide decisions on when and where to deploy AI effectively. (Sources: “A Clinician’s Wishlist for Healthcare AI Model Evaluations by Sarah Gebauer, MD, “Organizational Governance of Emerging Technologies: AI Adoption in Healthcare”.)
1️⃣0️⃣ Tell Me How You’re Going to Use My Data: As an AI vendor, are you planning to package my prescription patterns into a dataset to resell to insurers? Most ethical frameworks suggest patients should be informed if their data is being used to train AI systems. However, the guidelines for clinicians are much less clear. Be transparent about how you intend to use the data you collect. (Sources: “A Clinician’s Wishlist for Healthcare AI Model Evaluations by Sarah Gebauer, MD, “Organizational Governance of Emerging Technologies: AI Adoption in Healthcare”.)
1️⃣1️⃣ AI’s Overconfidence - Forcing Doctors to Second-Guess Themselves: “The unwavering confidence with which AI delivers its conclusions makes it incredibly difficult for a human to step back and say, ‘Hold on, I need to question this,’” says Dr. Wendy Dean, president and co-founder of Moral Injury of Healthcare, an organization dedicated to advocating for the well-being of physicians. (Source: Politico.)
1️⃣2️⃣ Fear That AI Would Replace Doctors in Decision Making and Make Doctors Less Relevant and Less Productive
81% of clinicians believe AI could “erode critical thinking”. (Source: The Deep View Report.)
77% say AI tools have made them less productive while increasing their workload. (Sources: The Register, Dr. Jeffrey Funk on LinkedIn.)
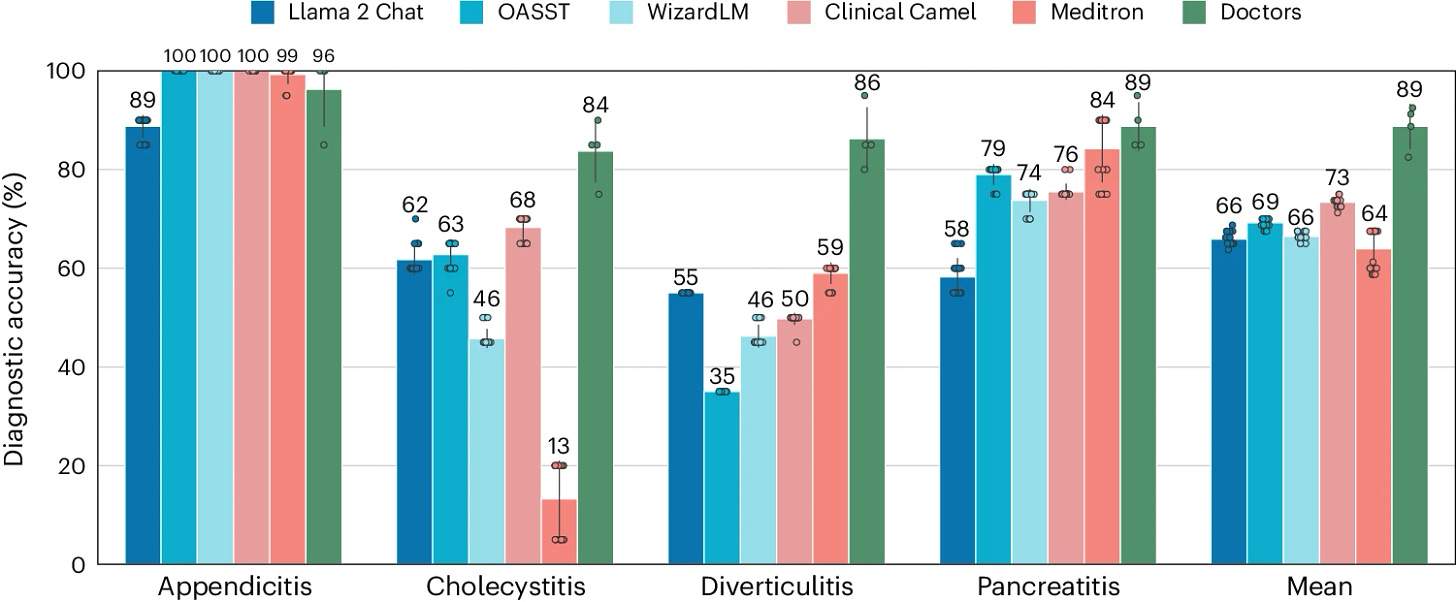
LLMs are currently not ready for autonomous clinical decision-making. While this is the conclusion of a recent paper in Nature Medicine, Dr. Braydon Dymm, MD argues on X, “While I agree that last year’s models could not match clinicians on autonomous clinical decision-making, I think that properly deployed systems utilizing the latest models (even open source Meerkat 70B) could give clinicians a run for their money! It remains to be proven.”
While some of these are valid concerns, others are myths.
So where do we stand in the “Who gets blamed when AI screws up in healthcare?” debate?
🔵 Doctors and Hospitals: Currently, doctors and hospitals are held responsible for patient care. If they use AI tools, they are expected to make the final decisions and ensure the AI’s recommendations are appropriate. (Source: Politico.)
🔵 AI Developers: There is also an argument that AI developers should be held accountable, especially if the AI system fails due to a flaw in its design or programming. (Source: Unite.ai.)
🔵 Legal and Regulatory Framework: The legal system is still catching up with these advancements. Courts and lawmakers are working, but working extremely slowly, to define the parameters of liability for AI in healthcare. (Sources: Politico, Stanford University Human Centered Artificial (HAI).)
🔵 Enterprise Liability: If a healthcare facility develops its own AI algorithms and removes human oversight, it could be held liable for any mistakes through the concept of enterprise liability. (Source: Future of Work News.)
🔵 Guidelines Urgently Needed: Patient and physician advocacy groups and other stakeholders are advocating for clearer guidelines and protections for doctors using AI, to ensure they are not unfairly penalized for relying on these tools. (Source: Politico.)
So with all these risks and uncertainties, you think doctors will just dive headfirst into the AI hype? Not a chance.
You can’t play the game if no one even knows the rules.
So, until someone defines the playbook, don’t hold your breath for AI to take over healthcare.
Conclusion
The tragic irony? AI in medicine could revolutionize healthcare, but until we nail down who’s on the hook when AI screws up, the fear is real: Doctors go to jail. Engineers don’t.
Doctors are wary, and for good reason. Right now, AI developers are cashing in on the upside while doctors are left holding the liability bag. Until the rules are written in stone, AI adoption in healthcare will crawl at a snail’s pace.
AI Liability Reform isn’t just a nice-to-have. It’s essential. Yet, there's been silence from Congress—not a single bill proposed. It’s time to call your representative. Don’t wait until after the election. If history tells us anything, “after the election” could mean waiting a long time. 😉
Hi! I believe in fair compensation for every piece of work. So, to those who’ve stepped up as paid subscribers: thank you. 🙏 If you’re not a paid subscriber yet and you want my relentless research and analyses like this one to continue for another 12 months, 24 months, 36 months, and more, consider joining the ranks:
👉👉👉👉👉 My name is Sergei Polevikov. I’m an AI researcher and a healthcare AI startup founder. In my newsletter ‘AI Health Uncut’, I combine my knowledge of AI models with my unique skills in analyzing the financial health of digital health companies. Why “Uncut”? Because I never sugarcoat or filter the hard truth. I don’t play games, I don’t work for anyone, and therefore, with your support, I produce the most original, the most unbiased, the most unapologetic research in AI, innovation, and healthcare. Thank you for your support of my work. You’re part of a vibrant community of healthcare AI enthusiasts! Your engagement matters. 🙏🙏🙏🙏🙏